Cyber Valley Research Fund treibt Exzellenz in der Forschung voran
Verstehen von grundlegenden Muster in Netzwerken
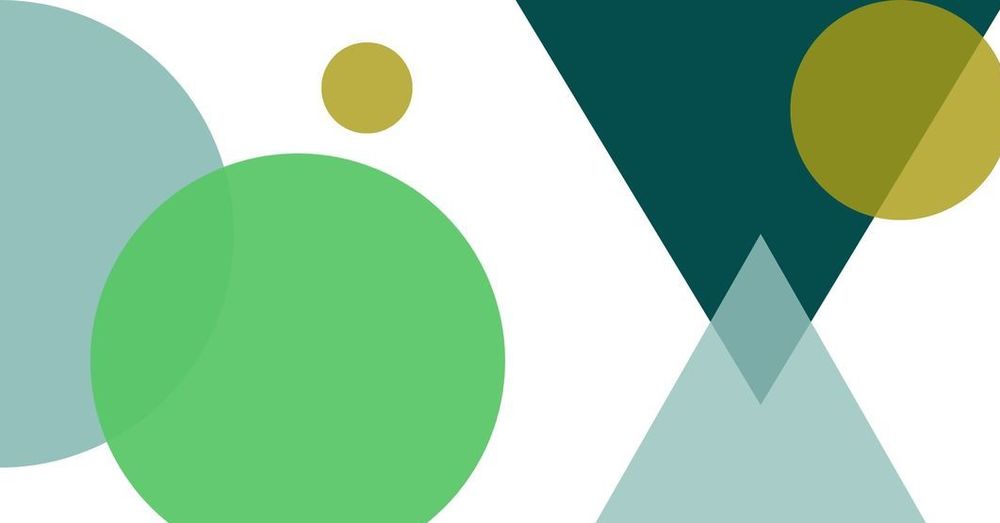
Six of Cyber Valley’s founding industrial partners – Amazon, BMW, Bosch, IAV, Porsche, and ZF Friedrichshafen – established the Cyber Valley Research Fund to support research projects that combine academic and industrial interests.
One notable project supported by the Research Fund was Caterina De Bacco’s project “Relaxing Restrictive Independence Assumptions in Networks”. The project aimed to improve our understanding of fundamental patterns in networks through advanced probabilistic generative models. It began in April 2020 and concluded in December 2023.
By leveraging the growing availability of rich datasets, De Bacco’s project developed advanced network models to capture complex interactions within various domains. The project team created a model that combines node information with network structure to analyze multilayer networks. By developing analytical approximations, the project addressed the complexity of real-world interactions, incorporating features like reciprocity and multiplexity.
Extending beyond the concept of conditional independence, the research team developed two new methods for modeling connections between nodes. One uses Poisson distributions and relies on pseudo-likelihood approximations, while the other utilizes Bivariate-Bernoulli distributions. Both methods provide a better understanding of network interactions.
Additionally, the project delved into how the developed ideas and methods could be applied to generalized data sets, specifically hypergraphs, where the interactions involve groups of nodes, and further developed their generative models. These models present several advantages for hypergraph analysis and can effectively identify communities and predict missing connections.
These new models represent a major advancement in network analysis, offering reliable and scalable solutions for understanding complex interactions. The project has set the stage for future research and two more models are currently in development, focusing on multilayer networks with additional information and hierarchical structures.
This project yielded the following publications:
-
M. Contisciani, E. A. Power, and C. De Bacco. “Community detection with node attributes in multilayer networks”. Scientific Reports 10.1 (2020), page 15736.
-
K.Higham, M. Contisciani, and C. De Bacco. “Multilayer patent citation networks: A comprehensive analytical framework for studying explicit technological relationships”. Technological Forecasting and Social Change 179 (2022), page 121628.
-
H. Safdari, M. Contisciani,and C. De Bacco. “Generative model for reciprocity and community detection in networks”. Physical Review Research 2 (2021).
-
M. Contisciani, H. Safdari, and C. De Bacco. “Community detection and reciprocity in networks by jointly modelling pairs of edges”. Journal of Complex Networks 10.4 (2022).
-
H. Safdari, M. Contisciani, and C. De Bacco. “Reciprocity, community detection, and link prediction in dynamic networks”. Journal of Physics: Complexity 3.1 (2022).
-
C. De Bacco, M. Contisciani, J. Cardoso-Silva, H. Safdari, G. Lima Borges, D. Baptista, T. Sweet, J.-G. Young, J. Koster, C. T. Ross, R. McElreath, D. Redhead, and E. A. Power. “Latent network models to account for noisy, multiply reported social network data”. Journal of the Royal Statistical Society Series A: Statistics in Society 183 (2023).
-
H. Safdari, M. Contisciani, and C. De Bacco. “Anomaly, reciprocity, and community detection in networks”. Physical Review Research 5.3 (2023).
-
M. Contisciani, F. Battiston, and C. De Bacco. “Inference of hyperedges and overlapping communities in hypergraphs”. Nature Communications 13.1 (2022).
-
N. Ruggeri, M. Contisciani, F. Battiston, and C. De Bacco. “Community detection in large hypergraphs”. Science Advances 28 (2023).
-
Q. F. Lotito, M. Contisciani, C. De Bacco, L. Di Gaetano, L. Gallo, A. Montresor, F. Musciotto, N. Ruggeri, and F. Battiston. “Hypergraphx: a library for higher-order network analysis”. Journal of Complex Networks 11.3 (2023).
Zugehörige Artikel
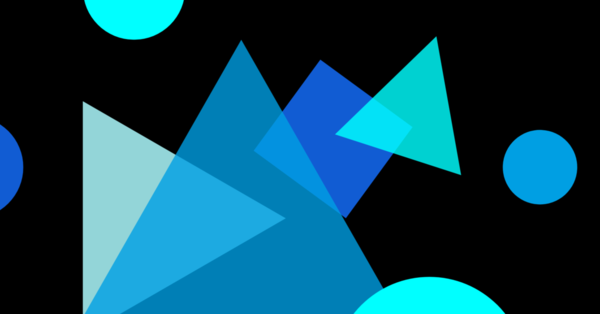
Cyber Valley Research Fund bringt robotische Wahrnehmungs...

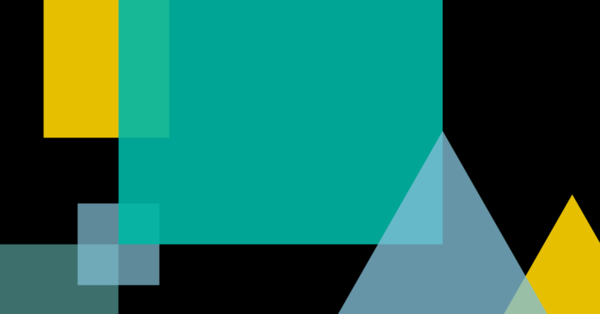
Cyber-physical twins of human organs

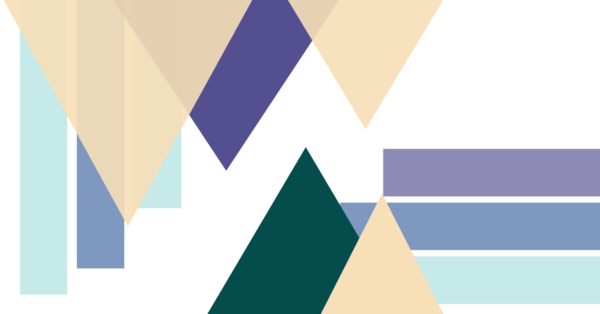
Der selbstüberwachte Roboter – erfolgreicher Abschluss ei...
